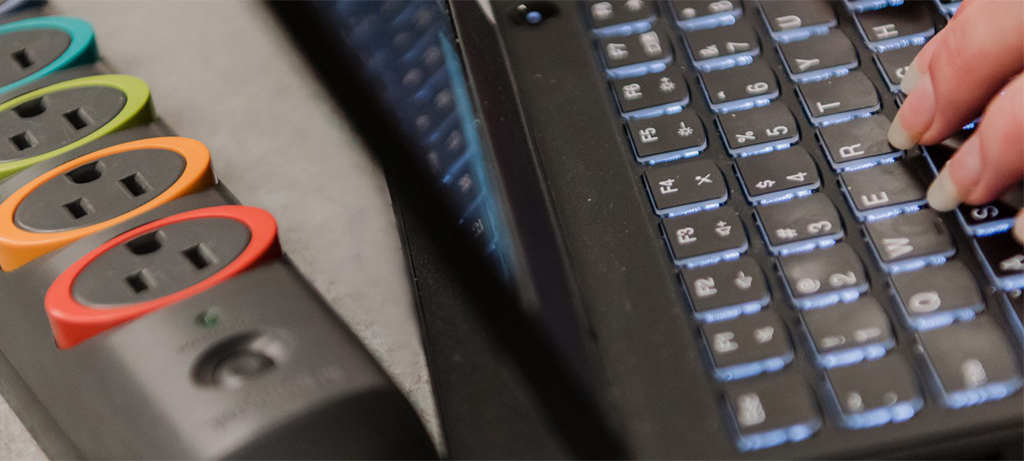
Two NSF awards will enhance data science courses, research, and student experiences
Data science has rapidly grown at UMBC, and faculty are now working to enhance data science courses, research, and student experiences. The goal is to ensure they are inclusive, empowering, and effective in preparing students to tackle the urgent problems our society is working to solve, and can scale up to meet student and workforce demands.
Faculty in both information systems, and computer science and electrical engineering have recently received two grants from the National Science Foundation to conduct research toward this aim.
Making data science more inclusive
NSF awarded funding to a team of researchers at UMBC; the University of California, Berkeley; and Mills College in California through the Improving Undergraduate STEM Education program. This funding will support the Undergraduate Data Science at Scale project at UMBC, including the development and implementation of a unique data science education program for undergraduate students in STEM and non-STEM disciplines, says Vandana Janeja, associate professor and chair of information systems (IS).
The data science field is relatively new as compared to the more established computing education, Janeja explains, and there are very few studies examining how these topics are taught to students. “This project will generate new knowledge about a data science curriculum and pedagogy designed to promote learning among diverse undergraduate students, many from groups underrepresented in STEM,” she says.
This novel approach to teaching data science will also “empower students as generators of new knowledge rather than passive recipients of existing information,” Janeja explains.
Another component of the project is the data scholars program, which will include students from traditionally underrepresented groups in STEM fields.
With these changes, Janeja anticipates that data science at UMBC will continue to expand. She’s working with UMBC’s Division of Information Technology to explore how UMBC, and other universities, will need to adapt and scale up offerings to meet the changing needs of students over time. “The findings will drive a community transformation in undergraduate data science education that can scale with student demand, and ultimately broaden participation in data science across multiple and diverse institutional settings.”
Janeja and UMBC colleagues are excited to develop a model that can have a nationwide impact, bringing new students into the field, and shaping how they approach work in data science. She expects that this work will set a foundation for colleges across the country looking to implement data science programs and better support the learning of data science students.
High-impact, team-based student research
Outside of the classroom, undergraduate students in computer science, information systems, and business technology administration will have the opportunity to work with government agencies in Baltimore City to tackle real challenges through a new NSF-funded program. Aryya Gangopadhyay, professor of information systems, has received support for the new program through the Data Science Corps under NSF’s Harnessing the Data Revolution (HDR) initiative.
HDR is one of NSF’s 10 “Big Ideas”: bold, visionary, national-scale activities to open up new frontiers in science and engineering. This program allows researchers to answer fundamental questions through new modes of data-driven discovery, Gangopadhyay explains. On this project, he will work with colleagues and students to collect and analyze data for projects that seek to improve Baltimore residents’ quality of life.
Gangopadhyay will partner with UMBC faculty including Anupam Joshi, professor and chair of computer science and electrical engineering (CSEE); Tim Oates, professor of CSEE; Nirmalya Roy, associate professor of IS; and Sanjay Purushotham, assistant professor of IS. The UMBC team will collaborate with faculty at Bowie State University, Towson University, and the University of Baltimore. Gangopadhyay and his team will work with UMBC’s Faculty Development Center to evaluate student learning outcomes for this project.
“The goal of the project is to develop a team-based data science program for undergraduate students in computing,” explains Gangopadhyay. Both undergraduate and graduate students will contribute to this research, gaining hands-on experience with the complexity of addressing urban infrastructure challenges, such as traffic congestion.
Students will also examine a range of ethical considerations, including data privacy, as they process information. Street sensors, for example, can collect sensitive information on peoples’ patterns of daily life. Students will come to better understand their role as researchers in protecting privacy, and other ethical considerations, as they cull through the data, says Gangopadhyay.
“Data science is poised to change the world by improving the quality of life through smart technologies,” explains Gangopadhyay. “Our students will play a part in bringing about some of these changes. Through their projects, students will develop analytical and coding skills and learn how to collaboratively work in real life projects with industry, government and academia, under the guidance of faculty mentors.”
Adapted from a UMBC News article by Megan Hanks. Banner image: A student using a computer. Photo by Marlayna Demond ’11 for UMBC.