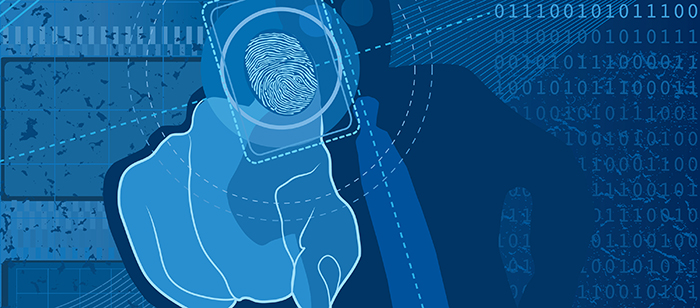
Dissertation Defense
Digital Forensics for
Infrastructure-as-a-Service Cloud Computing
Josiah Dykstra
10:00am Tuesday, 16 April 2013, ITE 325b
We identify important issues in the application of digital forensics to Infrastructure-as-a-Service cloud computing and develop new practical forensic tools and techniques to facilitate forensic exams of the cloud. When investigating suspected cases involving cloud computing, forensic examiners have been poorly equipped to deal with the technical and legal challenges. Because data in the cloud are remote, distributed, and elastic, these challenges include understanding the cloud environment, acquiring and analyzing data remotely, and applying the law to a new domain. Today digital forensics for cloud computing is challenging at best, but can be performed in a manner consistent with federal law using the tools and techniques we developed.
The first problem is understanding how and why criminal and civil actions in and against cloud computing are unique and difficult to prosecute. We analyze a digital forensic investigation of crime in the cloud, and present two hypothetical case studies that illustrate the unique challenges of acquisition, chain of custody, trust, and forensic integrity. Understanding these issues introduces legal challenges which are also important for federal, state, and local law enforcement who will soon be called upon to conduct cloud investigations.
The second problem is the lack of practical technical tools to conduct cloud forensics. We examine the capabilities for forensics today, evaluate the use of existing tools including EnCase and FTK, and discuss why these tools are incapable of trustworthy cloud acquisition. We design consumer-driven forensic capabilities for OpenStack, including new features for acquiring trustworthy firewall logs, API logs, and disk images.
The third problem is a deficit of legal instruments for seizing cloud-based electronically-stored information. We analyze the application of existing policies and laws to the new domain of cloud computing by analyzing case law and legal opinions about digital evidence discovery, and suggest modifications that would enhance cloud the prosecution of cloud-based crimes. We offer guidance about how to author a search warrant for cloud data, and what pertinent data to request.
This dissertation enhances our understanding of technical, trust, and legal issues needed to investigate cloud-based crimes and offers new tools and techniques to facilitate such investigations.
Committee: Dr. Alan T. Sherman (Chair), Dr. Charles Nicholas, Dr. Richard Forno, Dr. Simson Garfinkel (Naval Postgraduate School), Mr. Donald Flynn, JD (Department of Defense Cyber Crime Center)