Computer Science and Electrical Engineering
University of Maryland, Baltimore County
Data Analytics for Sustainability
Professor Katharina Morik
TU Dortmund University, Germany
11:00am-12:30pm, Thursday 22 May 2014, ITE 456, UMBC
Sustainability has many facets and researchers from many disciplines are working on them. Particularly knowledge discovery always considered sustainability an important topic (e.g., special issue on data mining for sustainability in Data Mining and Knowledge Discovery Journal, March 2012).
- Environmental tasks include risk analysis concerning floods, earthquakes, fires, and other disasters as well as the ability to react to them in order to guarantee resilience. The climate is certainly of influence and the debate on climate change received quite some attention.
- Energy efficiency demands energy-aware algorithms, operating systems, green computing. System operations are to be adapted to a predicted user behavior such that the required processing is optimized with respect to minimal energy consumption.
- Engineering tasks in manufacturing, assembly, material processing, and waste removal or recycling offer opportunities to save resources to a large degree. Adding the prediction precision of learning algorithms to the general knowledge of the engineers allows for surprisingly large savings.
Global reports on the millennium goals and open government data regarding sustainability are publicly available. For the investigation of influence factors, however, data analytics is necessary. Big data challenges the analysis to create data summaries. Moreover, the prediction of states is necessary in order to plan accordingly. In this talk, two case studies will be presented. Disaster management in case of a flood combines diverse sensor data streams for a better traffic administration. A novel spatiotemporal random field approach is used for smart routing based on traffic predictions. The other case study is in engineering and saves energy in the steel production based on the multivariate prediction of the processing end-point by the regression support vector machine.
Further reading:
- Katharina Morik, Kanishka Bhaduri, Hillol Kargupta “Introduction to Data Mining for Sustainability”, Data Mining and Knowledge Discovery Journal, Vol. 24, No.2, pp. 311 – 324, 2012.
- Nico Piatkowski, Sangkyun Lee, Katharina Morik “Spatio-Temporal Random Fields: Compressible Representation and Distributed Estimation”, Machine Learning Journal Vol.93, No. 1, pp: 115-139, 2013.
- Jochen Streicher, Nico Piatkowski, Katharina Morik, Olaf Spinczyk “Open Smartphone Data for Mobility and Utilization Analysis in Ubiquitous Environments” In: Mining Ubiquitous and Social Environments (MUSE) workshop at ECML PKDD, 2013.
- Norbert Uebbe, Hans Jürgen Odenthal, Jochen Schlüter, Hendrik Blom, Katharina MorikA novel data-driven prediction model for BOF endpoint. In: The Iron and Steel Technology Conference and Exposition in Pittsburgh (AIST), 2013.
- Alexander Artikis, Matthias Weidlich, Francois Schnitzler, Ioannis Boutsis, Thomas Liebig, Nico Piatkowski, Christian Bockermann, Katharina Morik, Vana Kalogeraki, Avigdor Gal, Shie Mannor, Dimitrios Gunopulos, Dermot Kinane, “Heterogeneous Stream Processing and Crowdsourcing for Urban Traffic Management” Procs. 17th International Conference on Extending Database Technology, 2014.
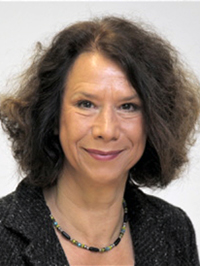
Katharina Morik is full professor for computer science at the TU Dortmund University, Germany. She earned her Ph.D. (1981) at the University of Hamburg and her habilitation (1988) at the TU Berlin. Starting with natural language processing, her interest moved to machine learning ranging from inductive logic programming to statistical learning, then to the analysis of very large data collections, high-dimensional data, and resource awareness.
Her aim to share scientific results strongly supports open source developments. For instance, RapidMiner started out at her lab, which continues to contribute to it. She was one of those starting the IEEE International Conference on Data Mining together with Xindong Wu, and was chairing the program of this conference in 2004. She was the program chair of the European Conference on Machine Learning (ECML) in 1989 and one of the program chairs of ECML PKDD 2008. She is in the editorial boards of the international journals “Knowledge and Information Systems” and “Data Mining and Knowledge Discovery”. Since 2011 she is leading the collaborative research center SFB876 on resource-constrained data analysis, an interdisciplinary center comprising 12 projects, 19 professors, and about 50 Ph. D students or Postdocs.