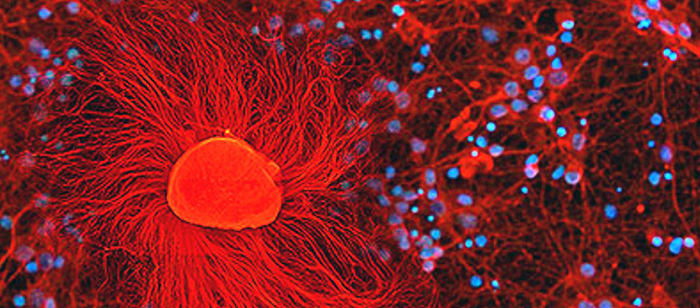
UMBC CSEE Seminar
A Neural Circuit Deconvolution Approach to Study Motivated Behavior
Dr. Joseph Cheer
Associate Professor, Deptartment of Anatomy and Neurobiology
and Department of Psychiatry
School of Medicine, University of Maryland Baltimore
11:001am-12:00pm, Wednesday, 3 February 2016, ITE 325b
Associate Professor, Deptartment of Anatomy and Neurobiology
and Department of Psychiatry
School of Medicine, University of Maryland Baltimore
In order to examine relationships between subsecond dopamine signaling and nucleus accumbens (NAc) cell firing during reward-directed behaviors, the ideal experimental approach is to record postsynaptic neuronal firing from the same electrode used to measure dopamine release. We have demonstrated that these measurements are feasible using cylindrical carbon fiber electrodes that can voltammetrically detect the oxidation potential of dopamine and also measure single-units extracellularly or local field potentials (LFPs). Moreover, we have added iontophoresis barrels to the carbon-fiber microelectrode to allow localized, rapid drug delivery to examine the signal transduction utilized by postsynaptic neurons when dopamine release is detected. The drugs to be ejected out of the iontophoresis barrels are selected on the basis of effects of prior microinjections (such as dopamine receptor antagonists). Once a significant behavioral effect is observed following the microinjection, iontophoresis pipettes with the same compounds are loaded for ejection in other animals. Under these conditions ongoing behavior is unaltered allowing for a detailed neurobiological dissection of the particular microanatomical domain during specific times of the behavioral sequence. Finally we can now provide causality between the two simultaneously recorded measures, by applying the above mentioned to animals amenable to optogenetic interrogation of dopaminergic pathways. We will show that dopamine sculpts cue-related patterned neuronal activity as well as the power of the NAc LFP during reward seeking.
Dr. Joseph Cheer graduated from Universidad de los Andes (Bogota, Colombia) with a B.S in Biology and Mathematics in 1996. He joined the Laboratory of Neurobiology and Experimental Microsurgery at the Colombian Neurology Foundation where he worked for 1 year investigating CNS regeneration using oncogene-tranfected cells and sciatic nerve co-grafts in motor cortex-lesioned animals. Joe received his Ph.D from The University of Nottingham (Neuroscience Section of the School of Biomedical Sciences) under the direction of Profs Charles Marsden and Dave Kendall and Dr Rob Mason. Dr. Cheer’s graduate research focused on the behavioral and electrophysiological effects of cannabinoids.
Dr. Cheer’s first postdoc (2000-2002) was spent in Sam Deadwyler’s laboratory (Wake Forest University School of Medicine) where he conducted research on multiple single-unit electrophysiology in freely moving organisms. Joe joined Mark Wightman’s lab as a post doc in fall 2002 at the University of North Carolina (Chapel Hill). There, he established the use of a microelectrode that allows for the simultaneous measurement of single-unit activity and dopamine release via fast-scan cyclic voltammetry.
Dr. Cheer is currently a tenured associate professor at the University of Maryland School of Medicine, where he directs several NIH and private foundation-funded graduate and undergraduate projects related to several neurophysiological and neurochemical aspects of endogenous cannabinoid signaling in intact systems.