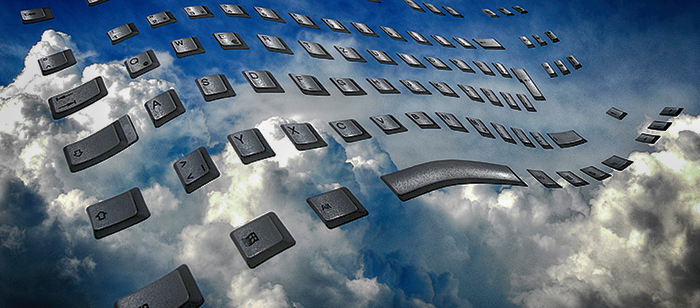
Rethinking the Cloud for Next-generation Applications
Tian Guo, University of Massachusetts
11:00am Monday, 21 March 2016, ITE325b
11:00am Monday, 21 March 2016, ITE325b
Today’s cloud platforms serve an increasing number of requests from millions of mobile users. This mobile workload introduces new challenges and workload dynamics that differ from traditional workloads. In the future, billions of Internet-of-Things (IoT) devices will connect to cloud platforms and compete for cloud resources. Current cloud platforms are agnostic to the type of end-devices and are not well suited to emerging application needs. My work argues that these trends require a rethinking of current cloud platforms and focuses on the challenges of handling the dynamics introduced by these next-generation applications.
In this talk, I will describe two aspects of cloud design: handling demand-side dynamics from emerging cloud workloads and handling supply-side dynamics from varying cloud platform resources. Specifically, I will describe model-driven mechanisms to optimize user-perceived performance for global workloads that exhibit spatial variations, and mechanisms to effectively support running applications on transient servers—servers with unpredictable availability. Finally, I will conclude my talk with future work in cloud research to handle emerging mobile and IoT applications.
Tian Guo is a Ph.D. student in the College of Information and Computer Sciences at University of Massachusetts Amherst. Her research interests include distributed systems, cloud computing, mobile computing and cloud-enabled IoTs. Her current focus is on handling dynamics introduced by new cloud workloads and emerging cloud platforms. She received her B.E. in Software Engineering from Nanjing University, China in 2010 and her M.S. in Computer Science from University of Massachusetts Amherst in 2013.